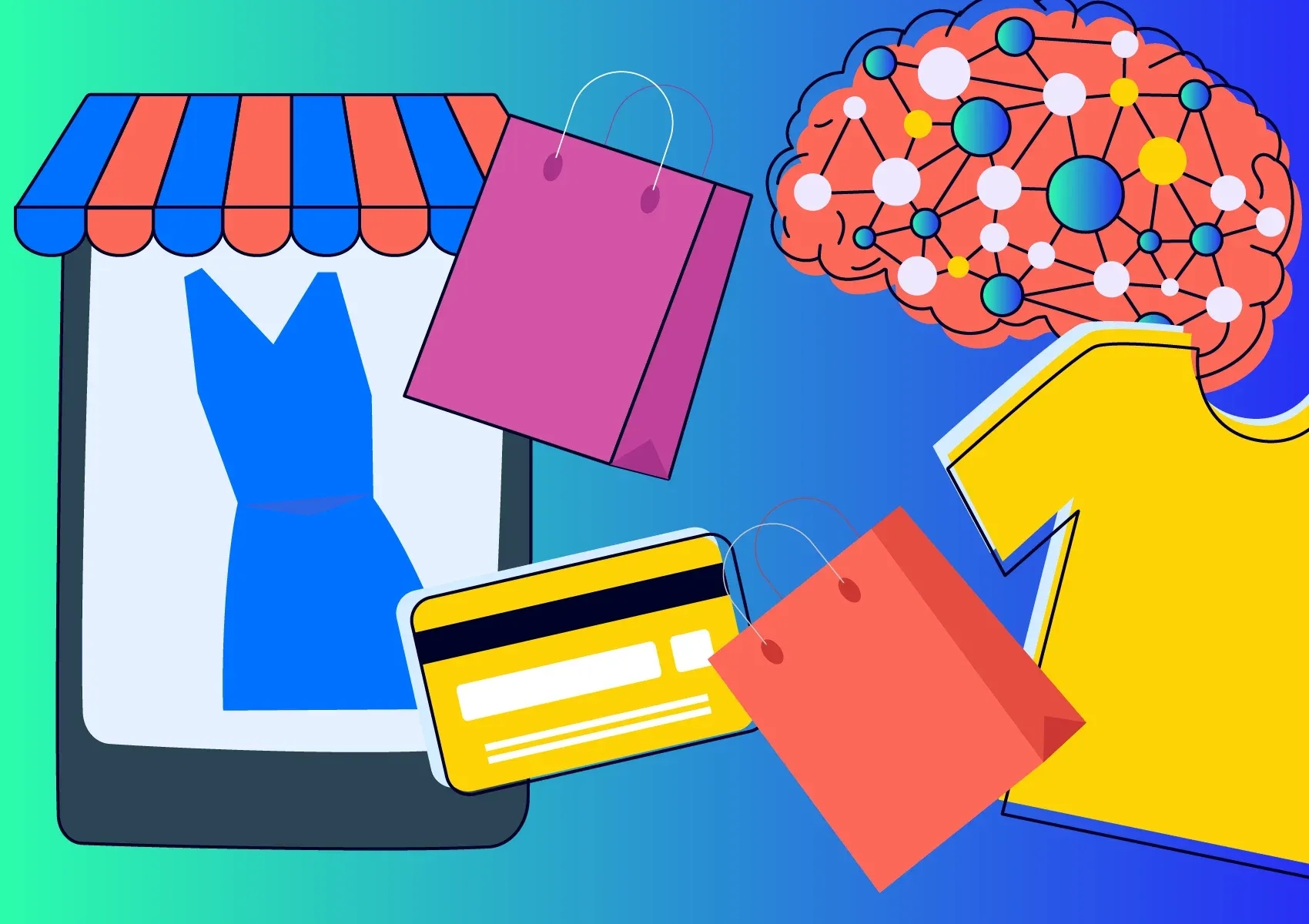
Are you struggling to keep up with inventory demands, losing sales to out-of-stock items, or dealing with costly overstock? This is where machine learning for ecommerce comes into play.
By integrating ML into your ecommerce operations, you can tune your inventory management, enhance search accuracy, detect and prevent fraud, and deliver highly personalized customer experiences.
So, in this article, we’ve gathered the best ways ML can help eCommerce businesses thrive by:
- Improving customer experience through personalization and targeted marketing
- Enhancing operational efficiency with predictive analytics
- Optimizing inventory to meet demand without overstocking
- Boosting security by detecting fraud in real-time
Business Benefits of Machine Learning in E-commerce in Numbers
1. Personalized Recommendations
2. Dynamic Pricing
3. Improved Search Results
4. Fraud Detection and Prevention
5. Inventory Management
6. Sentiment Analysis
7. Visual Search
8. Predictive Analytics
Summing up
FAQ
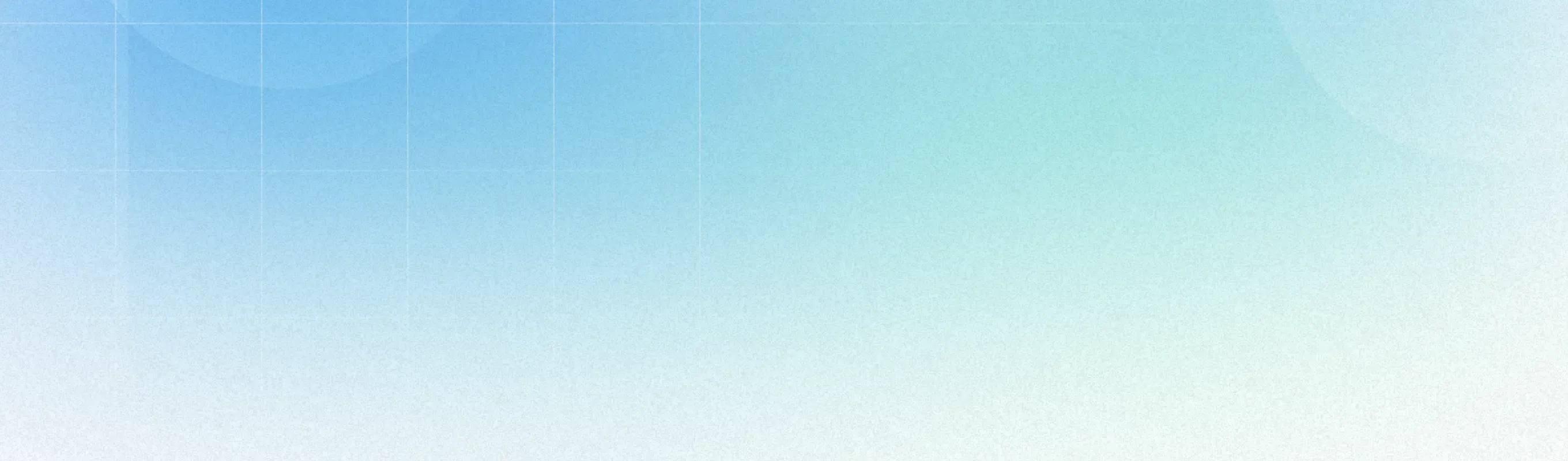
Learn how Onix built an Al-powered solution to find safe beauty products easily
Business Benefits of Machine Learning in E-commerce in Numbers
From Onix's experience, machine learning (ML) in e-commerce is a game-changer, driving measurable growth and efficiency. Here’s how our ML-powered solutions have brought real business benefits:
- Increased Sales by 20-30%: Personalization engines we've implemented deliver tailored recommendations, helping clients increase conversion rates and average order values.
- Customer Retention Up by 25%: With ML-driven segmentation and targeted campaigns, one client saw retention rates rise significantly by reaching the right audience with the right message.
- Reduced Operational Costs by 15-20%: Through ML-powered inventory management, e-commerce platforms can better forecast demand, reducing both overstock and stockouts.
- 40% Faster Fraud Detection: Our fraud detection systems use ML to flag suspicious transactions in real-time, minimizing revenue loss and enhancing customer trust.
These results show that integrating ML into e-commerce strategies can yield substantial returns, enhancing both revenue and operational efficiency.
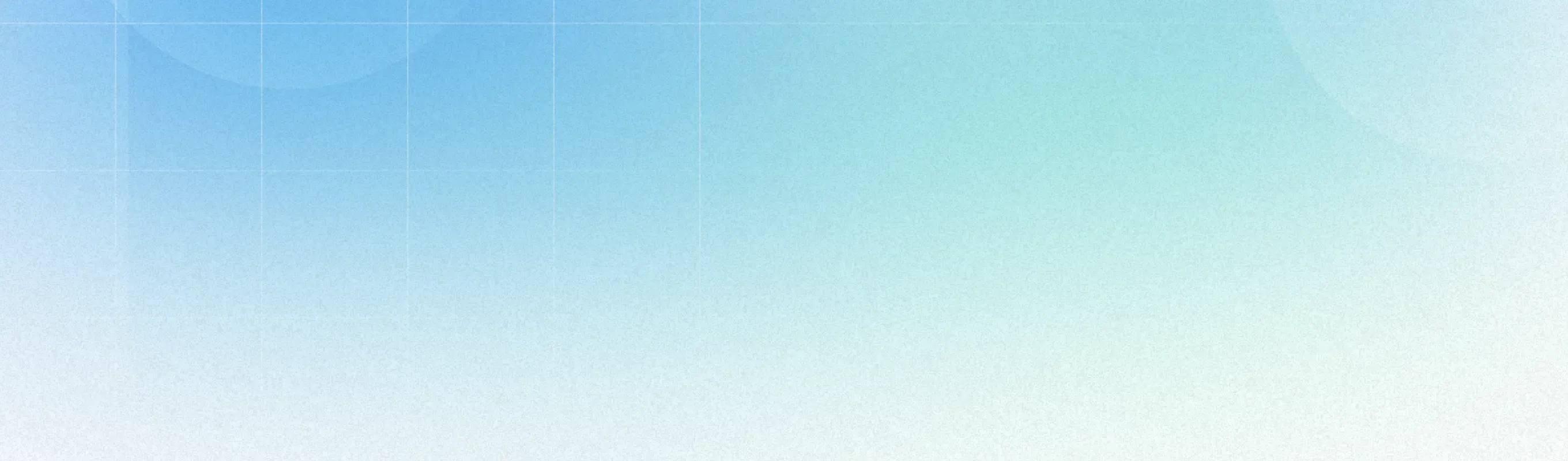
Unlock groundbreaking AI/ML solutions and drive business outcomes
Personalized Recommendations
"The ability to suggest relevant products based on individual preferences is the cornerstone of modern eсommerce. It transforms shopping into a personalized experience, driving both engagement and sales."
- Oleksandr Hergardt, Head of ML department.
Picture this: you're scrolling through your favorite online store, and suddenly, you see a list of products that seem like they were handpicked just for you. How does that happen? Well, that's machine learning for ecommerce at work!
Its algorithms are like super-smart detectives. They analyze tons of data - like your browsing history, past purchases, and even how much time you spend checking out certain products.
Then, they use all that info to figure out what you might like and suggest products tailored specifically to your tastes.
Take Amazon, for example. Their recommendation engine is a total game-changer. Did you know it's responsible for a whopping 35% of their sales? That's huge!
Amazon's algorithm uses different techniques like collaborative filtering and content-based filtering to make those spot-on suggestions.
So, if you're a tech geek who loves gadgets, you can bet Amazon will show you the latest and greatest devices and accessories that you're sure to drool over.
And it's not just Amazon getting in on the action. Netflix also uses ai and machine learning in ecommerce to up their recommendation game. Ever notice how they always seem to know exactly what you're in the mood to watch?
That's because they're analyzing your viewing habits and suggesting shows and movies that they think you'll love. The Netflix AI model continuously learns from user interactions to refine recommendations and enhance content discovery.
Dynamic Pricing
Dynamic pricing is like the chameleon - it changes and adapts in real-time based on what's happening in the market.
So, imagine you're shopping online for a new pair of shoes. The price you see might not be the same as what someone else sees, and that's because dynamic pricing is at play.
This strategy is all about adjusting prices on the fly, depending on things like how much demand there is, what the competition is up to, and even the time of day.
It's like a high-stakes game of chess, where businesses are constantly tweaking their prices to stay ahead of the curve.
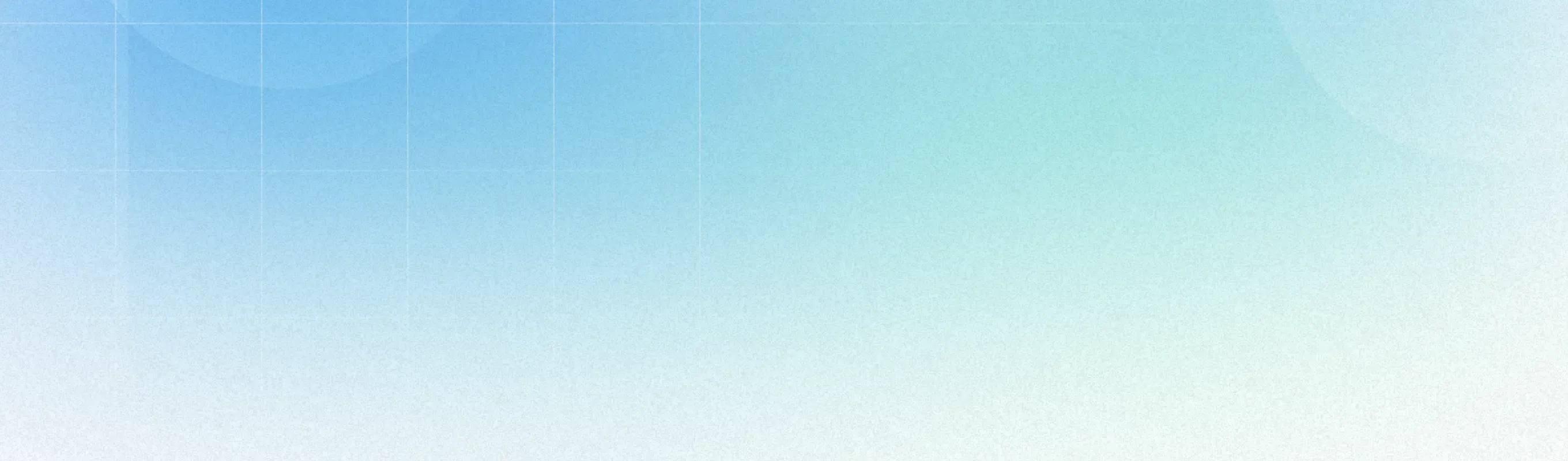
An Al-based solution for replacing faces in a video stream
Take airlines and hotels, for example. They've been using dynamic pricing for ages to adjust their rates based on demand and booking patterns.
So, if it's a busy holiday weekend and everyone is scrambling to book flights and hotel rooms, you might notice those prices creeping up. It's all about supply and demand!
But it's not just the travel industry getting in on the action. Ecommerce giants like Walmart and Amazon are using sophisticated algorithms to analyze market trends and adjust their prices accordingly.
So, if you're shopping for that new TV you've been eyeing, you might see the price drop if there's a sudden surge in competition, or it might go up if demand is through the roof.
Or, for example, Uber mastered the art of dynamic pricing, also known as surge pricing. Ever noticed how your ride costs more during rush hour or on a rainy day?
That's because Uber is adjusting fares based on real-time demand to ensure there are enough drivers on the road to meet your needs.
Improved Search Results
"Improved search functionality powered by machine learning helps customers find what they need quickly and efficiently, enhancing their overall shopping experience."
- Denis Sheremetov, Onix CTO
Alright, let's talk about how machine learning models for ecommerce are making search results way better in online shopping.
Have you ever typed something into a search bar, only to get a bunch of irrelevant stuff? Super frustrating, right? But ML in ecommerce helps to fix that.
It enhances search algorithms by really understanding what you're trying to find, even if your query is not relevant or has typos. This leads to more accurate and relevant search results, so you can find what you need faster.
Look at eBay, for instance. They use ML in ecommerce to boost their search accuracy. By analyzing how users behave and the feedback they give, eBay's algorithm can predict what you're after. This has made their search results 10-15% more relevant.
And then there's Google. They use an ML model called BERT (Bidirectional Encoder Representations from Transformers). BERT helps Google understand the context behind your search queries.
So, when you type something vague or even a bit messy, Google can still figure out what you mean and give you spot-on results.
Fraud Detection and Prevention
Now, let's move to security concerns. When you're shopping online, you want to feel secure, right? Well, machine learning is making online shopping a lot safer for everyone.
Recent research by PwC revealed that 51% of organizations have encountered fraud in the past two years, marking a 20-year high compared to previous surveys.
This surge in fraud primarily resulted in financial losses for these organizations. Many respondents emphasized the need for advanced technologies to combat this growing issue.
Additionally, some noted that the consequences of fraud extended beyond financial losses, causing operational disruptions and harming brand reputation and customer loyalty.
Ultimately, AI holds significant potential to help businesses maintain a secure payment environment, protecting their customers, revenue, and reputation.
ML algorithms analyze transaction patterns and detect any suspicious activity in real-time. This means they can spot fraud before it happens, protecting both businesses and customers.
Application of machine learning in ecommerce keeps an eye on transaction data. If they notice something fishy, like multiple transactions from different locations in a short period, they can flag it and take action.
This helps stop fraud in its tracks.
Stripe, another payment processing company, also uses machine learning in ecommerce to combat fraud. By analyzing user behavior and payment patterns, Stripe can detect and prevent fraudulent transactions.
It's like having a 24/7 security team watching over your online payments.
Read more about anomaly detection with ML in this article.
Inventory Management
"Machine learning use cases in ecommerce enables us to predict customer demand more accurately, ensuring businesses have the right products in stock at the right time."
- Oleksandr Hergardt, Head of ML department.
Let’s dive deeper. Imagine you're running an online store, and you need to keep popular items in stock without overloading on stuff that won't sell. That's why businesses should know how to use machine learning in ecommerce.
ML helps you predict demand and optimize inventory levels, so you're always stocked with what your customers want, when they want it.
Zara uses machine learning to predict fashion trends and manage their inventory smartly. This means they can quickly restock popular items and cut down on markdowns, keeping their shelves fresh and trendy.
Walmart is another great example. They use machine learning algorithms in ecommerce to analyze sales data and predict demand for their products. This helps them keep inventory levels just right, reducing waste and making sure they don't run out of hot items.
Now, let me tell you about a project we developed at Onix for one of our clients. We integrated a sophisticated ML system into their existing inventory management platform.
By analyzing past sales data, current market trends, and seasonal variations, our solution provided precise demand forecasts.
For instance, it identified that demand for certain electronics surged during the holiday season and recommended increased stock levels accordingly.
This not only minimize stockouts but also significantly reduced the costs associated with overstocking.
The client reported a 25% improvement in inventory turnover and a 15% reduction in holding costs, leading to higher efficiency and better customer satisfaction.
Sentiment Analysis
Now, let's talk about sentiment analysis. This is like giving businesses a superpower to understand what customers are really saying about their products and services.
Applications of machine learning in ecommerce analyze reviews and social media posts, businesses can get a clear picture of customer opinions and make improvements where needed.
Amazon, for instance, uses sentiment analysis to gauge how customers feel about their products. By diving into reviews, they can spot common issues and make data-driven decisions to enhance their products.
Coca-Cola uses sentiment analysis too, but they focus on social media. By monitoring mentions and feedback, they can quickly respond to negative comments and keep customers happy.
Visual Search
Let's dive into visual search, where finding products is as easy as snapping a photo. This feature is perfect for visually-driven shoppers who want to discover items online without typing a single word.
Take Pinterest's Lens feature, for example. Users can take a photo of an item, and Pinterest will find similar products on the platform. This not only drives engagement but also boosts sales by making shopping more fun and interactive.
ASOS is another cool example. They allow customers to upload photos of clothing they like and find similar items in their inventory. This makes the shopping process super intuitive and enjoyable.
Predictive Analytics
Next up, predictive analytics. This is where machine learning for e-commerce really shows off its crystal ball abilities, forecasting future trends, customer behavior, and sales. This helps businesses plan better and make informed decisions.
Starbucks uses predictive analytics to find the best spots for new stores. By analyzing demographic data and customer preferences, they can pinpoint areas with high potential for success.
Target also leverages predictive analytics to forecast inventory needs. This ensures they have the right products available when customers want them, reducing stockouts and overstock situations.
"Predictive analytics provides valuable insights into future trends and customer behavior, enabling businesses to stay ahead of the curve and meet customer needs more effectively." - Howard Schultz, Former CEO of Starbucks
With this knowledge, you can understand how ML is reshaping the industry and consider how it might enhance your own operations. The possibilities are exciting and endless!
Summing up
Machine learning technology in ecommerce is transforming ecommerce, addressing key challenges and enhancing the online shopping experience.
From personalized recommendations and dynamic pricing to improved search results and fraud detection, ML makes ecommerce smarter and more efficient.
By embracing machine learning use cases for ecommerce, businesses can better meet customer needs, optimize operations, and drive growth. Leaders like Amazon, Walmart, and innovative projects from companies like Onix demonstrate the immense potential of ML.
The future of ecommerce is bright with ML at its core, promising a more personalized, efficient, and secure shopping experience. Embrace the power of ML and unlock new opportunities for your business today.
Contact us for a free consultation about your ML idea.
FAQ
How does machine learning improve online e-commerce sales?
Machine learning enhances personalization, recommending products based on user behavior, improving customer experience, and optimizing pricing strategies, leading to higher conversion rates and sales.
How to use machine learning to optimize product search?
By implementing natural language processing (NLP) and collaborative filtering, machine learning can analyze search queries and user preferences to deliver more relevant search results and improve overall user experience.
How does machine learning help with inventory management in e-commerce?
Machine learning forecasts demand based on historical sales data, seasonal trends, and external factors, enabling better inventory control, reducing stockouts, and minimizing overstock situations.
Which machine learning technologies are best suited for e-commerce?
Technologies like recommendation engines, predictive analytics, natural language processing (NLP), and computer vision are particularly effective in enhancing user experience and operational efficiency in e-commerce.
How to implement machine learning in your online store?
Start by identifying key areas for improvement, gather and prepare relevant data, select appropriate machine learning models, integrate them into your platform, and continuously monitor and refine the system based on performance metrics.
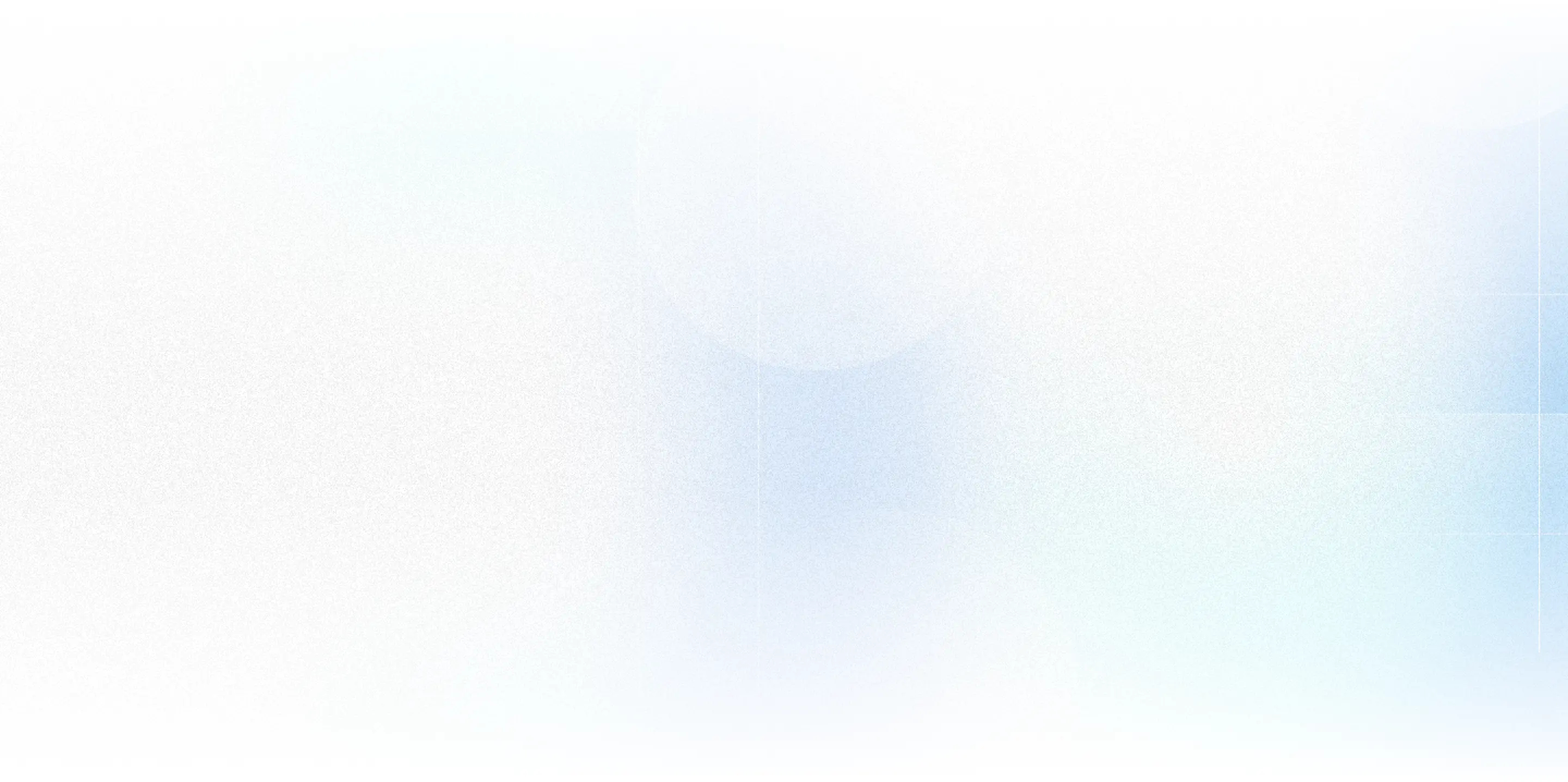
Never miss a new blog post from us!
Join us now and get your FREE copy of "Software Development Cost Estimation"!
This pricing guide is created to enhance transparency, empower you to make well-informed decisions, and alleviate any confusion associated with pricing. In this guide, you'll find:
Factors influencing pricing
Pricing by product
Pricing by engagement type
Price list for standard engagements
Customization options and pricing
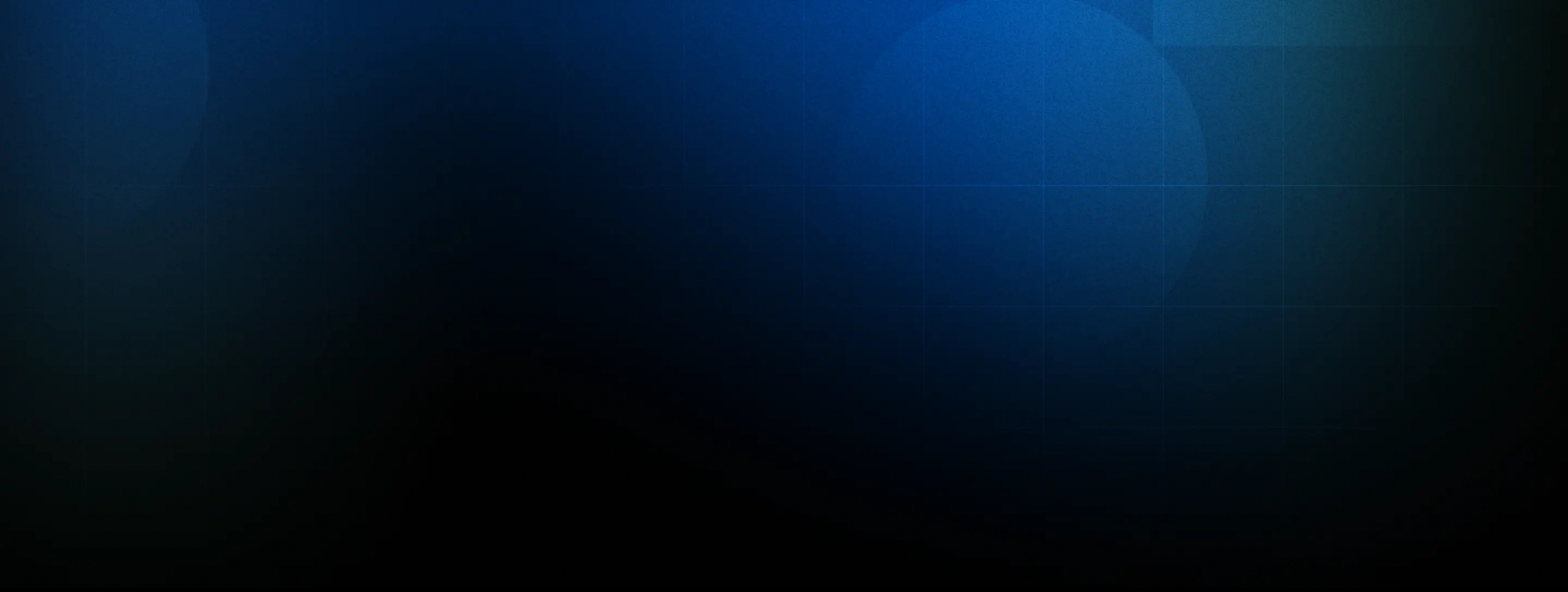