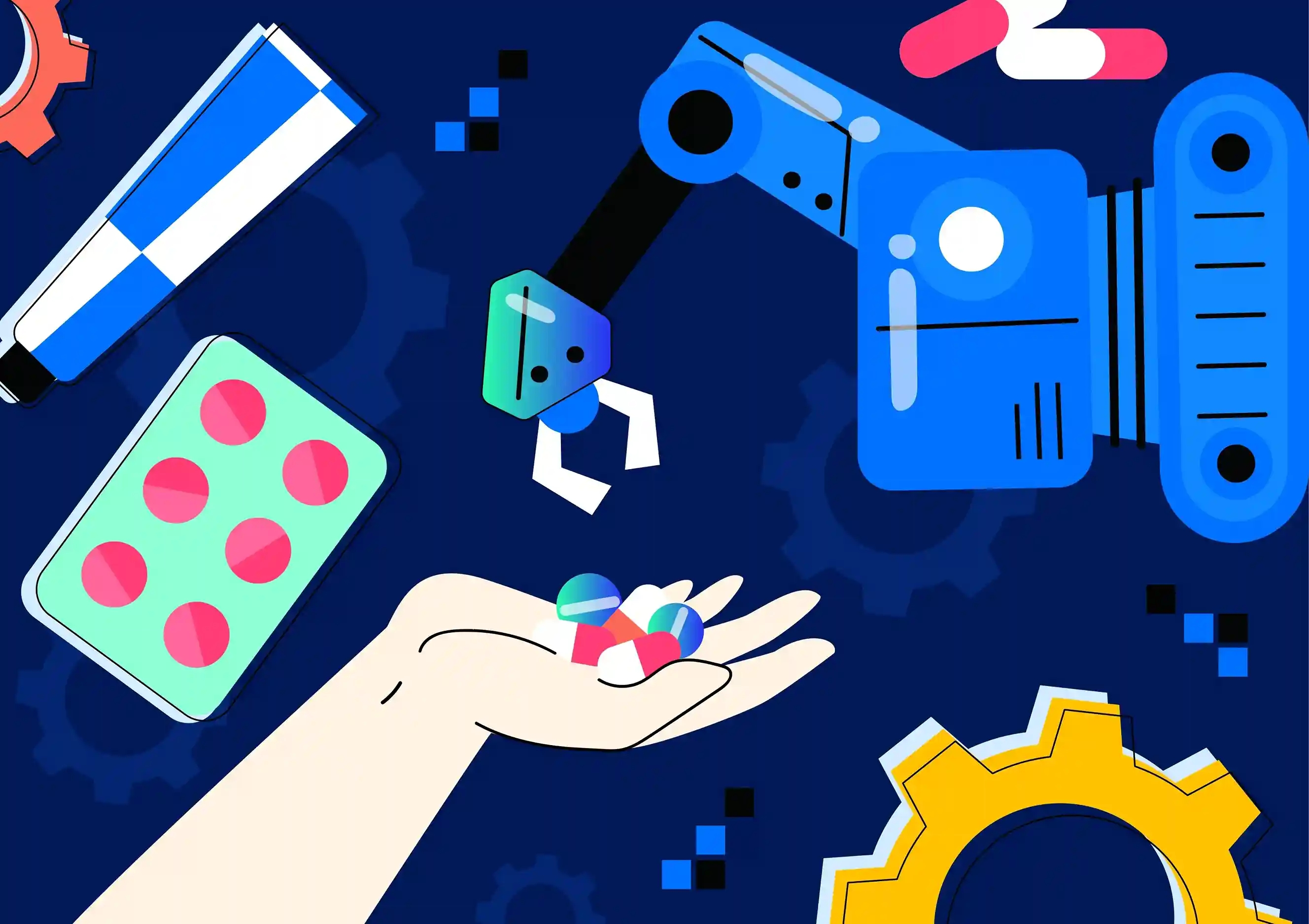
If you work in the pharmaceutical industry, you are probably asking yourself the following questions:
- How to reduce the time for drug development?
- Why are research costs so high?
- Why is finding the right molecule such a challenge as a needle in a haystack?
- And how to avoid inefficient R&D spending?
But what if we told you that there is an effective way to deal with these problems? Namely, implementing artificial intelligence in the pharmaceutical industry.
According to the McKinsey Global Institute, generative AI could contribute $60 billion to $110 billion to the pharmaceutical industry every year. How?
Mainly because it can:
- boost productivity
- accelerate the process of discovery of new drug compounds
- speed up development and approvals.
Generative AI for pharmaceuticals is already rewriting the rules of the industry.
Opportunities For Generative AI in Pharma: Stats and Facts
GenAI in Pharma: Key Use Cases You Need to Know
Strategies for Effective Implementation of AI in Pharma
Ready To Implement AI in Your Pharma? We Can Help at Any Stage
FAQ
Read on to learn about the top use cases of generative AI in pharma and how the technology can benefit your business.
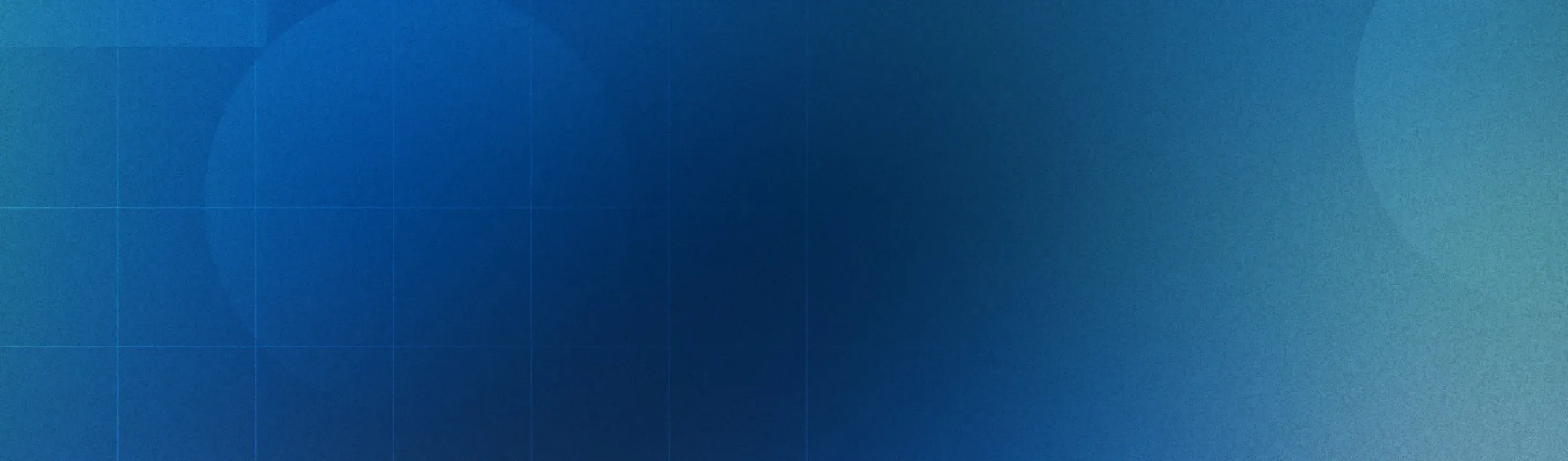
Book your free one-hour consultation and get your ML solution demo within a week!
Opportunities For Generative AI in Pharma: Stats and Facts
Generative AI will transform the pharmaceutical industry by creating significant opportunities across:
- drug discovery
- clinical trials
- productivity
- health outcomes.
Productivity improvement
Experts estimate GenAI has the potential to boost productivity by 30% and impact nearly 40% of life sciences work hours.
Here is what Gen AI can do:
- Less time spent on paperwork, as AI can handle the creation of detailed clinical trial documents, regulatory forms, and marketing materials.
- You get a personal research buddy who’s always ready to help. GenAI helps you to organize data, manage clinical trials, and suggest improvements.
- Smarter sales support since GenAI generates personalized scripts and offers real-time help during client meetings.
Accelerating drug discovery and development
Generative AI can analyze massive datasets and generate molecular designs, reducing the drug discovery phase from years to months.
On average, reducing drug development by even one day can save pharmaceutical companies up to $6.5 million.
McKinsey forecasts that generative AI could bring the pharmaceutical industry up to $110 billion in annual economic value.
Reduction of clinical trial costs
As practice shows, clinical trials are expensive and slow. Luckily, generative AI is making a big difference.
According to a BCG report, AI could save time and costs by at least 25%–50% in the stages of drug discovery leading up to the preclinical stage.
Gen AI can help pharmaceutical companies to:
- analyze medical histories and find the right patients for trials, reducing the dropout rates.
- accelerate data analysis by providing real-time insights that make decision-making more straightforward and more accurate.
Moving away from a one-size-fits-all approach
Generative AI can collect and organize huge amounts of data from different sources, which can help customize and personalize interactions (for example, to support direct sales and medical teams).
Moreover, generative AI can also adjust content to fit various cultural contexts and communication channels.
This adaptability benefits applications like:
- training,
- interactions with healthcare professionals,
- and patient support services.
Generative AI is expected to increase revenue by up to 10% and reduce external agency costs by 25% or more.
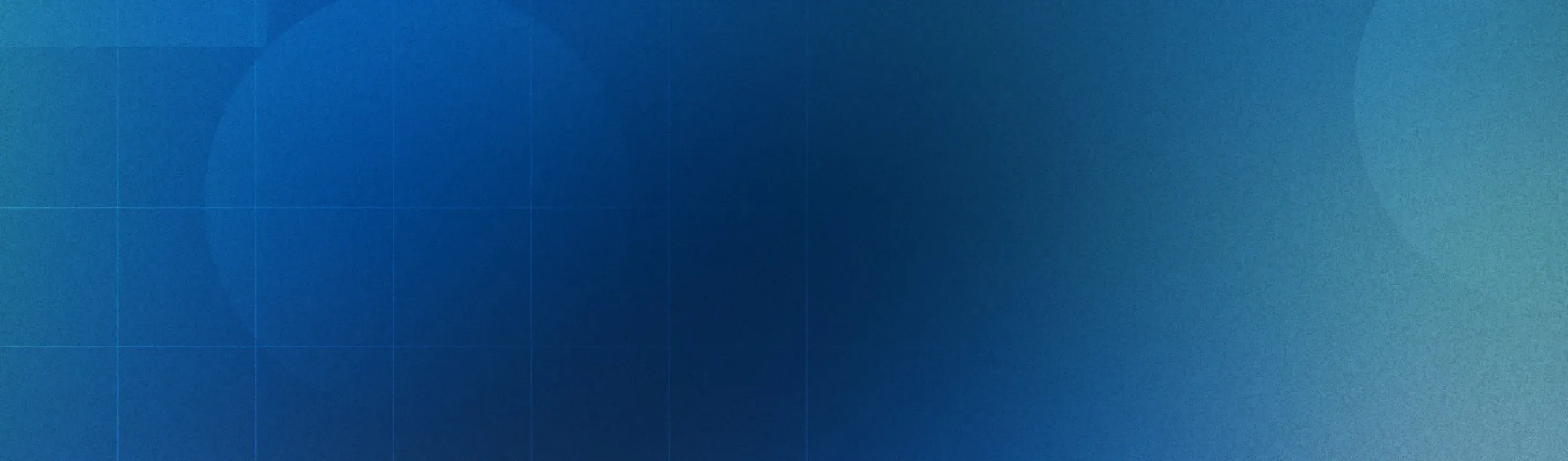
Learn how Onix built an Al-powered solution to find safe beauty products easily
GenAI in Pharma: Key Use Cases You Need to Know
Let's consider the key AI use cases in the pharmaceutical industry without further ado.
Drug discovery and development
More than half of the resources spent on drug development go to waste due to inefficient data use, leading to longer timelines and higher costs.
Generative AI can significantly speed up and optimize the process of drug discovery and development by streamlining data processes But that's not all. This technology can increase the chances of success in developing safe and effective treatments.
Let's consider how it all happens:
- Molecular design
Generative AI analyzes vast amounts of chemical and biological data to create new molecules.
Using deep learning models it can help you to:
- predict which molecular structures are most likely to become effective drugs
- focus on the most promising candidates.
This approach cuts the trial and error process by 25%, making drug discovery faster and more accurate. In addition, generative AI is expected to accelerate drug development further.
- Identifying drug candidates
AI can quickly pinpoint promising candidates by simulating how molecules interact with biological targets, cutting the discovery timeline from years to months.
- Repurposing existing drugs
For example, Insilico Medicine used machine learning algorithms to discover a new drug to treat lung disease.
Insilico used generative AI for each step of the preclinical drug discovery process:
- to identify a molecule that a drug compound can target
- to create new drug candidates
- to assess how well these candidates will connect with the target
- to predict the results of clinical trials.
Thanks to AI, they managed to do it at just 10% of the typical $400 million cost!
Clinical trials and research optimization
We all know that the development of new drugs requires a lot of time. To tell the truth, the whole process can last 12-15 years and cost more than $1 billion.
Fortunately, technology is evolving. Generative AI in the pharmaceutical industry is expected to:
Let's consider how it improves the process:
- Patient recruitment
By analyzing electronic health records, genetic information, and other data, AI can help you identify patientswho meet certain research criteria. This way, you can reduce recruitment times and drop-out rates, resulting in more efficient trials.
- Real-time data analysis
Generative AI accelerates data processing by automatically analyzing test data and generating insights. This allows researchers to make quick decisions, adapt protocols, and identify potential problems early.
- Documentation
Generative AI makes documentation easy by using natural language generation to generate protocols, test reports, and regulatory documents quickly.
It can save up to 30% of the time usually spent on writing medical documents, helping teams focus on other critical tasks while keeping processes efficient and stress-free.
- Trial monitoring
Generative AI-powered dashboards and alerts help teams track progress, predict delays, and adjust logistics in real-time, improving trial efficiency.
For example, Bayer Pharmaceuticals uses generative AI with tools like Google Cloud’s Vertex AI and Med-PaLM 2 to speed up drug development.
These AI solutions make it easier for researchers to:
- sift through massive datasets
- uncover meaningful patterns
- handle time-consuming tasks like drafting clinical trial documents and translating them into multiple languages.
Personalized medicine
Machine learning algorithms can analyze massive patient data to develop a personalized approach.
Generative AI technology shifts away from the "one-size-fits-all" model to focus on:
- a patient's unique genetic makeup
- lifestyle
- medical history.
This allows AI to:
- Analyze genetic data
Generative AI processes large datasets from genetic sequencing to identify patterns and mutations associated with specific conditions. This helps predict how patients respond to certain treatments, allowing for more accurate prescriptions.
- Design custom treatments
AI can suggest personalized drugs or dosages by modeling how different molecules interact with a person's genetic and biological profile.
- Monitor patients efficiently
Generative AI integrates data from wearable devices and EHRs to provide real-time information about treatment effectiveness, helping doctors adjust therapy on the fly.
- Support precision diagnostics
AI can analyze patient data together with clinical and imaging information to provide earlier and more accurate disease diagnoses.
Tempus uses artificial intelligence to personalize cancer treatment by analyzing tumor genetics along with big clinical data.
The company collects genetic information from patient samples, such as tumors, blood, and saliva, and integrates it with clinical records in its machine-learning platform.
This approach allows Tempus to discover patterns and correlations in huge data sets, helping oncologists determine the most effective treatment options for individual patients.
Inventory planning and optimization
Next-generation AI-powered planning tools analyze historical and market trends to:
- predict a sharp increase in demand
- anticipate bottlenecks and disruptions in supply chains
- create proactive intervention plans
- automatically control inventory
- make production plans in real-time, considering available materials, current customer demand, and operational constraints.
Based on the recent McKinsey research, using generative AI in supply chains can:
- reduce supply chain costs by 2-3%
- increasing the accuracy of inventory planning and forecasts by 15%
- reducing workload by 20-30% for demand planning specialists.
For example, Sanofi uses AI tools in its supply chain to predict inventory shortages. The system accurately predicts 80% of low-stock situations, allowing the company to quickly secure the necessary supplies and avoid disruptions.
Strategies for Effective Implementation of AI in Pharma
At Onix, we understand general AI's enormous opportunities for the pharmaceutical industry.
However, realizing this potential requires a careful assessment, planning, and execution strategy.
“Pharmaceutical companies should focus on areas where AI can make a real difference. This is the key to cutting through the hype, understanding what AI can actually achieve, and setting practical goals to maximize its impact.”
– Oleksandr Hergardt, Head of ML Department.
Below, we share our key strategies to ensure the successful adoption of AI in pharma while solving business challenges and maximizing returns on investment.
Set clear goals
Before integrating artificial intelligence, it is vital to identify the specific problems you want to solve or opportunities you wish to explore.
This may include such goals as:
- Acceleration of drug discovery
- Improving patient care through personalized medicine
- Optimization of clinical research processes
Read also: How to Move From Idea to Prototype [A Step-By-Step Guide]
At this stage, our specialists collaborate with you to conduct workshops and align AI capabilities with your business goals, delivering measurable results.
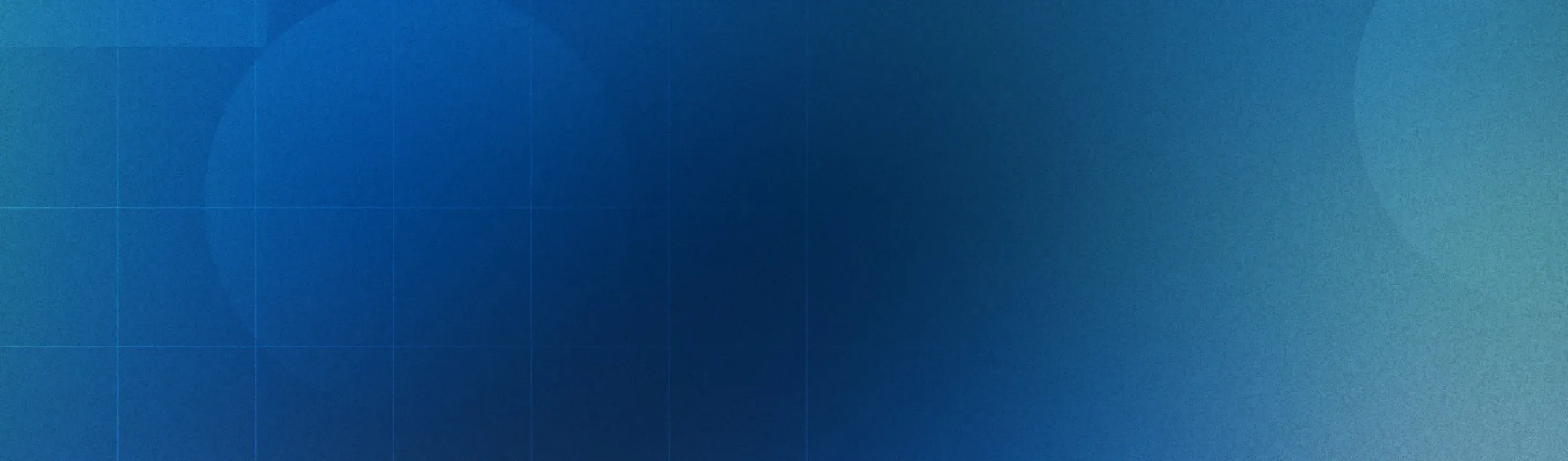
Gain valuable insight into your future product
Start with a proof of concept (PoC)
Starting with a PoC, you can test the viability of AI on a smaller scale.
"An AI PoC acts as a prototype that showcases potential functionalities and uncovers hidden challenges. It’s like a rehearsal before the grand performance, ensuring everything is in place."
– Oleksandr Hergardt, Head of ML Department.
How Onix can help at this stage:
- Defining the AI challenges you want to address ( we help you understand your pain points, determine your goals, and clarify how AI can drive value for your business.)
- Data preparation essentials for AI (we guide you on how to gather, prepare, and organize relevant data for your AI PoC.)
- Building the right AI solution (we help you decide whether to create a custom AI solution or use an existing one.)
- Evaluating the value potential of your AI PoC (we explore different evaluation metrics and methodologies to determine whether your PoC is delivering the desired outcomes.)
- Enhancing and scaling your AI proof of concept for optimal results (we guide you through strengthening and scaling your AI solution for optimal results.)
Read also: The Ultimate Guide to AI Proof of Concept
Use high-quality data
AI thrives on data, so its quality, quantity, and availability are critical.
Pharmaceutical companies often have large but fragmented datasets that need:
- cleaning
- integration
- labeling.
During this stage, our ML team provides data cleansing, standardization, and integration from various sources, such as EMRs, research datasets, and clinical trials.
Learn more: How to Build Teams for AI Projects
Choose an appropriate technology stack
With numerous AI platforms and tools available, choosing the right one for your needs is critical.
Our experts help you select the right technology stack to ensure:
- performance
- flexibility
- easy integration of functionality
- seamless user experience
- scalability
- interoperability with your existing systems.
Address regulatory and ethical issues
Generative AI has risks, such as:
- violation of intellectual property and copyright
- cybersecurity vulnerabilities and data privacy
- biased results
- opaque results and false results (known as hallucinations).
The good news is that the risks can be managed by implementing strong policies and guidelines for responsible AI use.
AI in pharma must comply with stringent regulatory requirements and ethical standards, such as GDPR for data privacy or FDA guidelines for AI in healthcare.
These safeguards should not just be rules. They should become a natural part of the company's culture and day-to-day operations.
At this stage, Onix specialists ensure that your AI solutions meet all regulatory standards by providing documentation and supporting compliance audits.
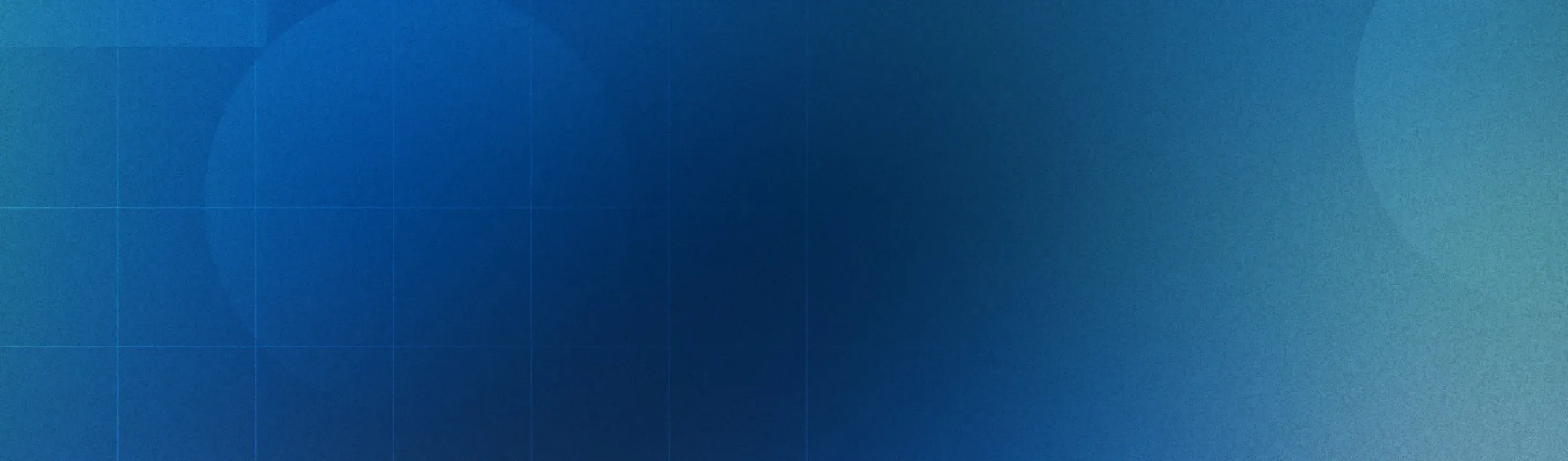
Ready To Implement AI in Your Pharma? We Can Help at Any Stage
Ready To Implement AI in Your Pharma? We Can Help at Any Stage
We’ve just scratched the surface of how generative AI in the pharmaceutical market can be used. This technology is just gaining momentum, and its possibilities are huge.
If you’re curious about how to leverage genAI for your business, we’re here to help.
Whether you’re exploring ideas or already working with AI, we offer:
- Consultations to clarify your vision
- Proof of concept to test feasibility
- Custom development to create solutions from scratch
- Improvements to enhance existing tools
- Seamless integration into your current processes
Let’s discuss your goals and turn them into actionable results!
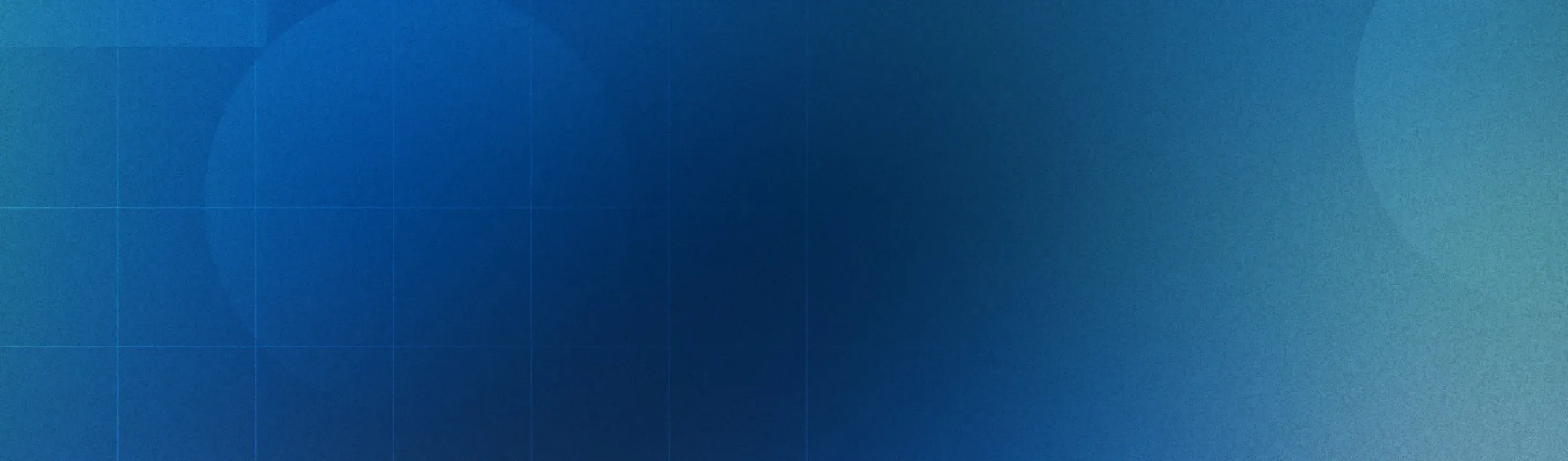
You have an app idea, and we have the expertise you need. Let's team up!
FAQ
Which companies are using AI for pharma the most?
Major companies like Pfizer, Novartis, and Johnson & Johnson use AI for drug discovery, clinical trial optimization, and predictive analytics. Small pharma companies also implement AI to enhance productivity and reduce costs.
For example, Insilico Medicine and Exscientia also implement AI to identify new drug candidates and streamline research processes. Cloud-based AI solutions and scalable tools make it accessible, even for companies with limited resources.
How can Onix help with AI implementation in the pharmaceutical industry?
Onix can help with AI implementation in the pharmaceutical industry by developing customized AI solutions that enhance operational efficiency, streamline data analysis, and automate processes.
With our expertise in AI, machine learning, and system integration, Onix can support pharmaceutical companies in leveraging AI to ensure smooth integration, reduce costs, and accelerate innovation.
How does generative AI in the pharmaceutical industry help discover new drug molecules?
Generative AI speeds up drug discovery by predicting molecular structures and generating new drug candidates based on data analysis. It can explore vast chemical spaces quickly, identifying promising compounds that might take humans years to find. This significantly accelerates the process of identifying potential drug molecules.
What challenges and risks are associated with implementing generative AI in pharma?
Implementing generative AI in pharma presents challenges, such as data privacy concerns, the need for high-quality, accurate data, and regulatory compliance. There are also risks related to AI model biases, transparency in decision-making, and integrating AI solutions into existing systems.
Implementing AI bias detection is essential to identify and mitigate potential biases, ensuring more reliable and ethical AI applications. These challenges require careful planning, robust data management, and collaboration with regulatory bodies.
In what ways can generative AI in the pharmaceutical industry improve clinical trial efficiency?
Generative AI can improve clinical trial efficiency by optimizing patient recruitment, analyzing medical data to identify suitable candidates, and predicting patient treatment responses. It helps identify suitable trial candidates faster and reduces trial duration, thus lowering costs and speeding up the time to market.
Additionally, generative AI for UX is transforming pharma by enhancing user interfaces for clinical dashboards, automating report generation, and personalizing digital experiences for researchers and healthcare professionals.
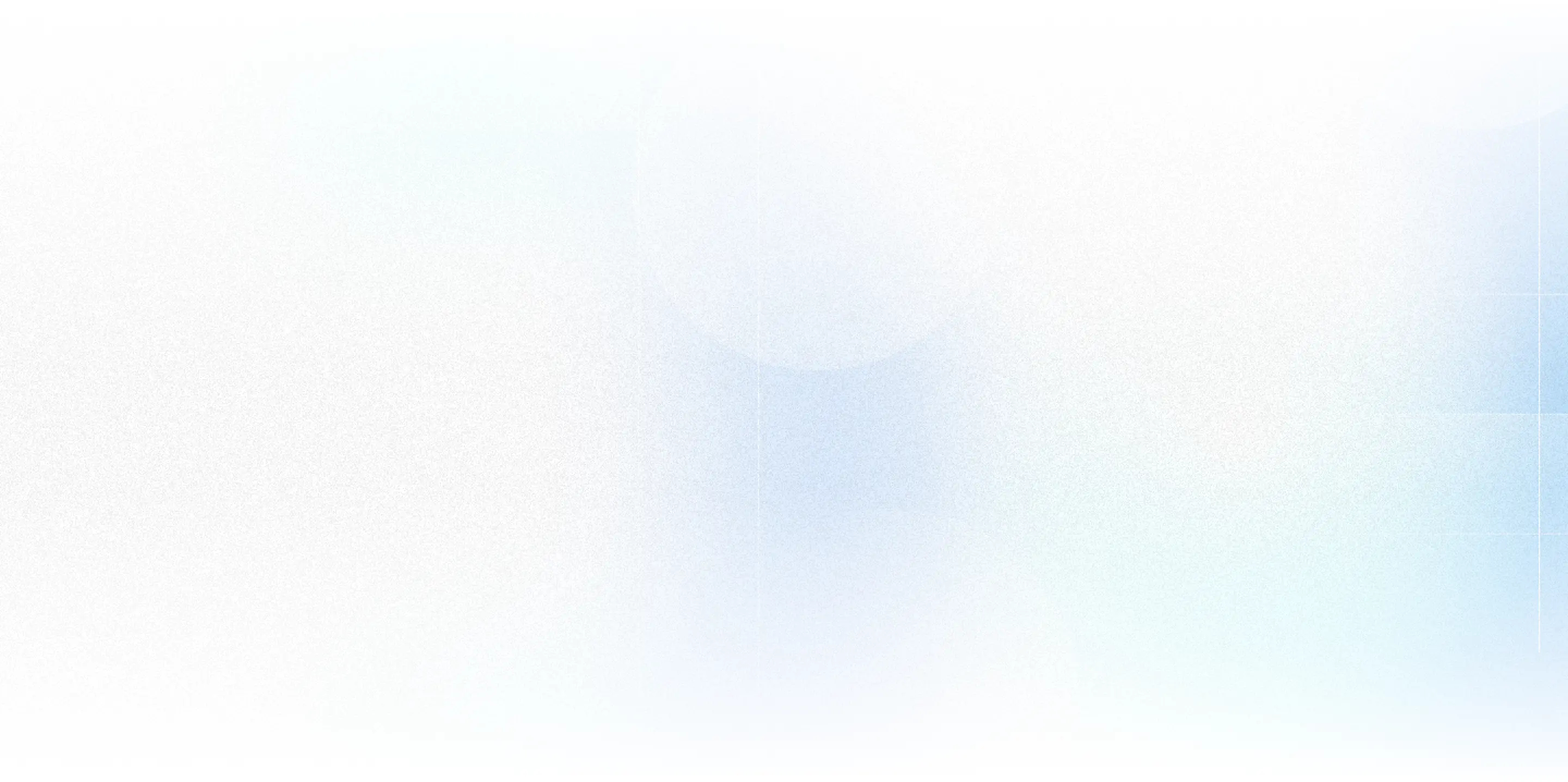
Never miss a new blog post from us!
Join us now and get your FREE copy of "Software Development Cost Estimation"!
This pricing guide is created to enhance transparency, empower you to make well-informed decisions, and alleviate any confusion associated with pricing. In this guide, you'll find:
Factors influencing pricing
Pricing by product
Pricing by engagement type
Price list for standard engagements
Customization options and pricing
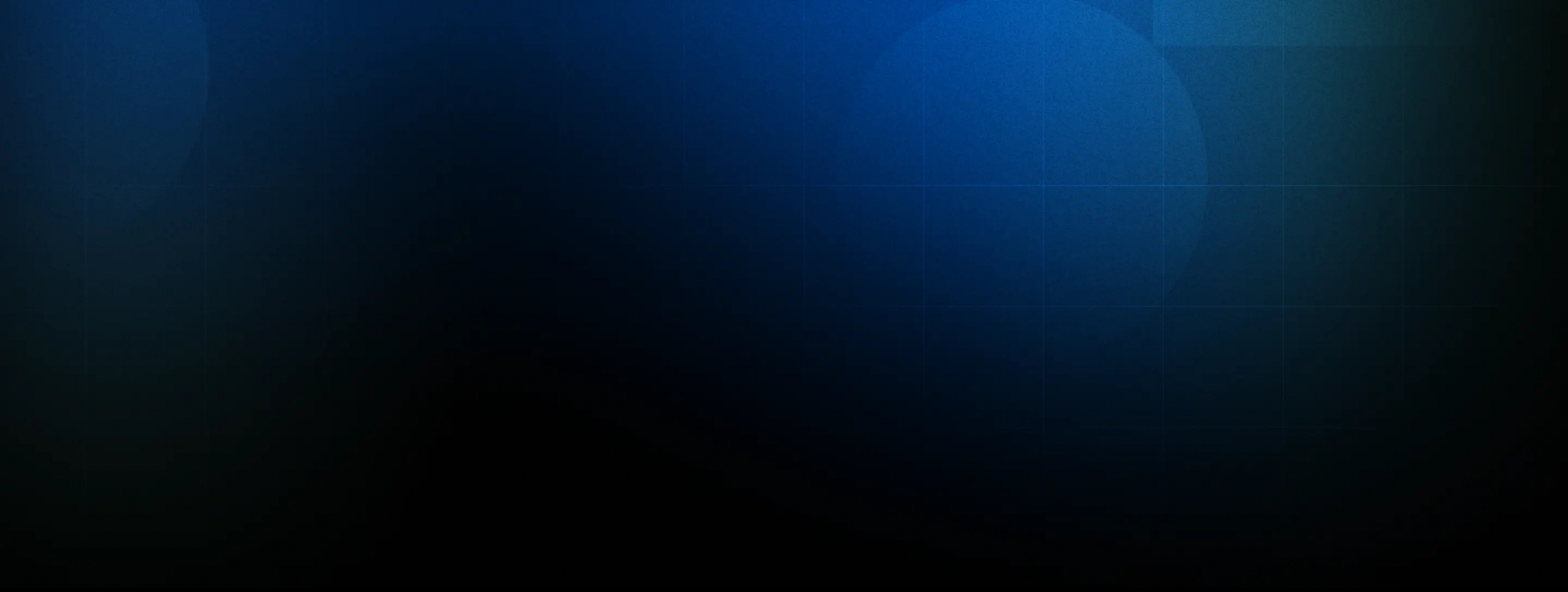