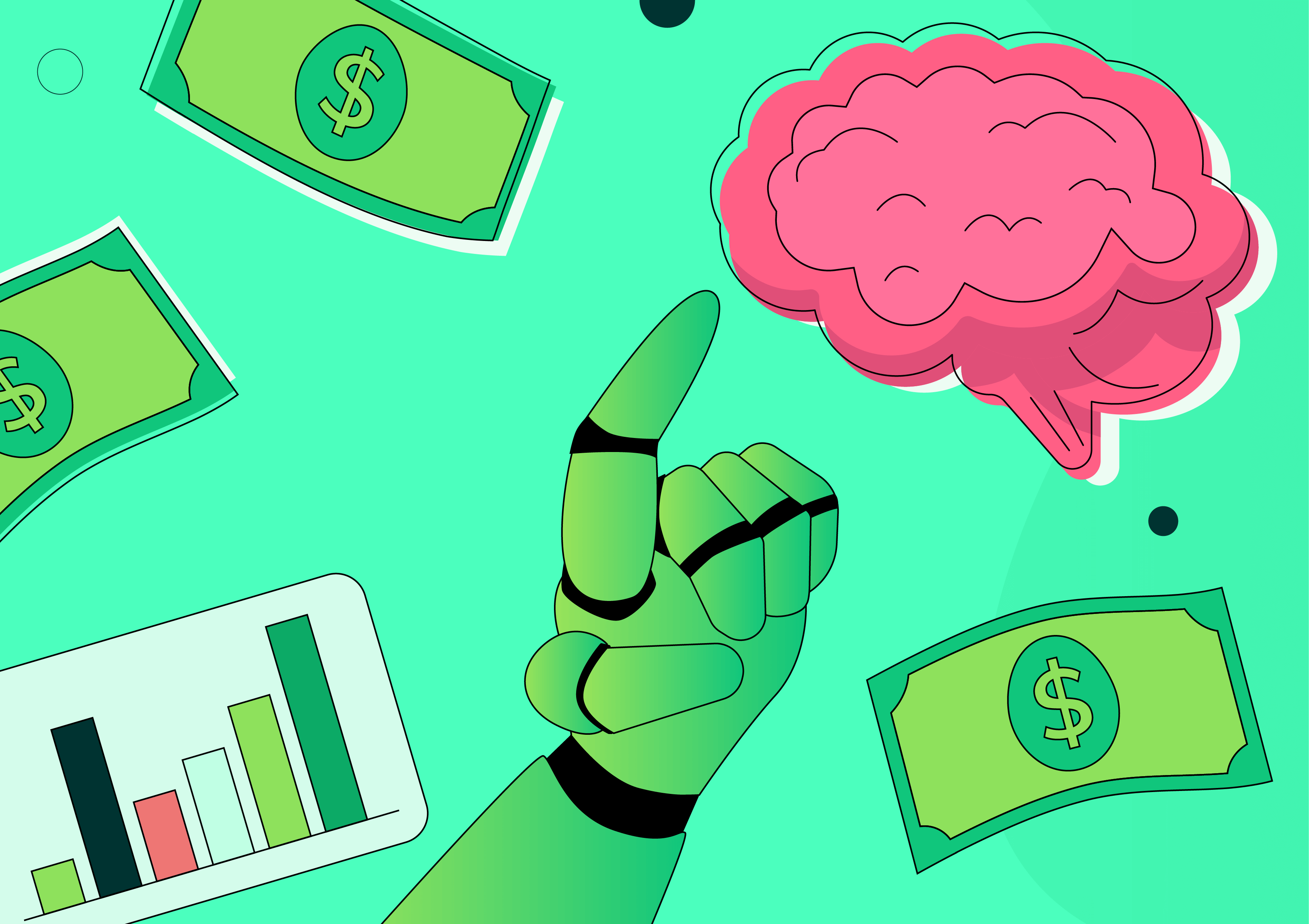
In today's technology-driven world, machine learning (ML) has emerged as a powerful tool for businesses to gain insights, automate processes, and make data-driven decisions. Machine Learning business applications are revolutionizing industries, offering advanced capabilities that were once considered out of reach.
At Onix, a renowned leader in the field of ML and AI solutions, our team of experts has accumulated extensive knowledge and experience in developing machine learning business applications.
In this article, we aim to:
- Provide a comprehensive overview of the pros and cons of ML business apps;
- Explore business applications of machine learning;
- Consider the challenges that organizations must consider.
What Are The Benefits of Applying ML Technology to Your Business?
Disadvantages of Machine Learning for Businesses
Applications of Machine Learning in Business From Market Leaders
Final Thoughts
FAQ
What Are The Benefits of Applying ML Technology to Your Business?
Machine learning technology offers numerous benefits to businesses across various industries. Here are eight key advantages of applying ML technology, along with examples and relevant statistics:
Improved Decision-Making
Machine learning algorithms can analyze vast amounts of data and identify patterns, leading to more informed and data-driven decision-making processes.
Example: A retail company can use ML to analyze customer purchasing patterns and behavior, enabling them to make personalized product recommendations. According to McKinsey, companies that use data-driven insights are 23 times more likely to acquire customers and six times more likely to retain them.
Enhanced Customer Experience
ML allows businesses to provide personalized customer experiences, increasing satisfaction and loyalty.
Example: An e-commerce platform can leverage ML to analyze customer preferences and browsing history, tailoring product suggestions to individual users. A study by Segment found that 44% of consumers are more likely to become repeat buyers after a personalized shopping experience.
Predictive Maintenance and Reduced Downtime
ML can be employed to predict equipment failures or maintenance needs, leading to reduced downtime and cost savings.
Example: A manufacturing plant can use ML algorithms to monitor machine sensor data and predict when maintenance is required. According to Deloitte, predictive maintenance can lead to a 12% reduction in scheduled maintenance costs and up to a 30% reduction in maintenance costs overall.
Optimized Supply Chain Management
ML can optimize inventory management, demand forecasting, and logistics, improving efficiency and cost savings.
Example: A logistics company can use ML to analyze historical data and predict demand fluctuations, allowing them to optimize warehouse inventory levels. According to a study by PwC, organizations that effectively use ML in their supply chain can reduce costs by up to 10% and increase revenue by up to 20%.
Fraud Detection and Risk Management
ML algorithms can analyze real-time transactions, identifying fraudulent or anomalous activities and minimizing risks.
Example: The travel industry can use ML to detect and prevent online travel fraud.
Automated Customer Support
ML-powered chatbots and virtual assistants can handle customer queries and issues, improving response times and customer satisfaction.
Example: An online service provider can deploy a chatbot to handle common customer inquiries, leaving the customer support team to focus on more complex issues. Juniper Research shows chatbots are expected to save businesses over $8 billion annually by 2023.
Targeted Marketing Campaigns
ML can analyze customer data to create targeted marketing campaigns, increasing conversion rates and ROI.
Example: An online advertising platform can use ML algorithms to identify the most relevant audience segments for a particular product or service.
Competitive Advantage
Embracing ML technology can provide a competitive edge in the market by enabling businesses to innovate and adapt quickly.
Example: A software development company integrating ML into its products gains a competitive advantage by offering cutting-edge features and functionalities. According to an MIT Sloan Management Review survey, 83% of companies agree that AI and ML are essential for their business's competitiveness.
By leveraging the power of ML, businesses can transform their operations, enhance customer experiences, and drive growth in a data-driven world. However, investing in the right expertise, infrastructure, and data quality is crucial to reap these benefits successfully.
Disadvantages of Machine Learning for Businesses
While machine learning (ML) offers numerous benefits, it is not always the best choice for every business scenario. Here are some of the top disadvantages of using machine learning in certain situations:
High Initial Investment and Ongoing Costs
Implementing machine learning models can be expensive, requiring specialized hardware, software, and skilled personnel. Additionally, ongoing maintenance and updates add to the costs.
Data Privacy and Security Concerns
Machine learning relies heavily on data, and businesses must handle sensitive customer information carefully. Data breaches or misuse can lead to severe legal and reputational consequences.
Complexity and Skill Gap
Developing and deploying ML models requires expertise in data science and advanced programming skills. Many businesses may lack the resources or skilled ML teams to implement solutions effectively. The solution to this challenge is to choose a trusted technology partner like Onix and be patient about team expertise.
Data Quality and Availability
Machine learning heavily depends on high-quality data. If the available data is insufficient, biased, or of poor quality, it can lead to inaccurate and unreliable predictions.
Overfitting and Generalization Issues
ML models may perform exceptionally well on training data but struggle to generalize to unseen data, leading to overfitting. Ensuring models generalize well to new data can be challenging.
Ethical and Bias Concerns
Machine learning models can inadvertently perpetuate bias in the training data, leading to unfair or discriminatory outcomes. Addressing bias in ML is a complex issue and requires careful consideration.
Lack of Interpretability
Some algorithms and machine learning techniques for business applications can be challenging to interpret. This lack of transparency can be problematic, especially in critical decision-making scenarios.
Limited Domain Knowledge
ML models lack human intuition and domain knowledge, which can lead to misinterpretations of context and incorrect conclusions in certain situations.
Dependency on Data Continuity
Machine learning models trained on historical data may not be effective if the underlying patterns or dynamics of the business change significantly.
Regulatory and Compliance Challenges
Specific industries have strict regulations regarding the use of data and ML models. Complying with these regulations can be burdensome and time-consuming.
Before applying machine learning to a business problem, it's essential to conduct a thorough analysis to determine whether the benefits outweigh the disadvantages. Sometimes, more straightforward methods or traditional analytics may be more suitable for achieving the desired outcomes.
Additionally, ethical considerations and data privacy should always be at the forefront of any ML implementation to ensure responsible and sustainable use of technology.
Applications of Machine Learning in Business From Market Leaders
Market leaders across various industries have successfully applied machine learning, leading to impressive outcomes. Here are some famous use cases where applying ML had excellent results:
Amazon: Personalized Recommendations
Amazon is a prime example of using machine learning for personalized product recommendations. By analyzing customer behavior, purchase history, and browsing patterns, Amazon's recommendation system suggests relevant products to users. This has significantly contributed to increased sales and improved customer satisfaction.
Read also: ML in Ecommerce
Google: Language Translation and Image Recognition
Google employs machine learning in its Translate and Photos services. Google Translate uses neural machine translation to provide more accurate and natural language translations. Additionally, Google Photos uses ML-based image recognition to categorize and tag images, making it easier for users to search and organize their photos effectively.
Netflix: Content Recommendations
Netflix leverages machine learning algorithms to suggest personalized content to its users. By analyzing viewing history, user ratings, and other behavioral data, Netflix's recommendation system helps users discover new shows and movies they will likely enjoy. It is estimated that up to 80% of the content watched on Netflix comes from personalized recommendations.
Facebook: Face Recognition and News Feed
Facebook utilizes machine learning in various ways. One prominent use case is face recognition, where ML algorithms can automatically tag users in photos. Facebook's news feed is personalized for each user based on their engagement patterns and interests, ensuring users see the most relevant content.
Uber: Dynamic Pricing and ETA Predictions
Uber employs machine learning to determine real-time dynamic ride pricing based on demand and supply. ML algorithms analyze historical ride data, traffic patterns, and other factors to accurately predict and display estimated time of arrival (ETA) for riders. This has helped Uber optimize pricing and enhance user experiences.
Airbnb: Search Ranking and Personalization
Airbnb uses machine learning to rank search results and personalize the user experience. The search ranking algorithm considers various factors such as property quality, pricing, location, and user preferences to display the most relevant listings to potential guests. Personalization extends to tailored emails and notifications, increasing user engagement and booking rates.
Tesla: Autonomous Driving
Tesla's self-driving technology relies heavily on machine learning and artificial intelligence. Tesla's Autopilot uses neural networks to analyze data from sensors and cameras, enabling the vehicle to navigate autonomously and make real-time driving decisions. ML is crucial in improving safety and enhancing autonomous capabilities over time.
IBM Watson: Healthcare and Diagnostics
IBM Watson, an AI-powered platform, has been applied in the healthcare industry for various purposes, including medical diagnostics and drug discovery. ML algorithms can analyze vast amounts of medical data, research papers, and patient records to assist in diagnosing diseases, recommending treatments, and accelerating drug development processes.
These are just a few examples of how machine learning has significantly impacted businesses and industries. ML's ability to process large volumes of data, identify patterns, and make predictions has improved efficiency, personalization, and innovation in various domains, making it an invaluable tool for market leaders worldwide.
Final Thoughts
In conclusion, integrating machine learning (ML) technology into businesses presents a double-edged sword with compelling advantages and significant challenges. On the positive side, ML offers improved decision-making capabilities, enhanced customer experiences, predictive maintenance, optimized supply chain management, and targeted marketing campaigns.
By harnessing the power of ML, market leaders like Amazon, Google, and Netflix have achieved remarkable success, driving revenue growth and customer loyalty through personalized recommendations and services.
However, businesses must be mindful of the potential drawbacks, including high initial investment costs, data privacy, and security concerns, bias issues, interpretability challenges, and the need for specialized skills.
While ML can deliver immense benefits and provide a competitive edge, its successful implementation requires an expert team to address ethical considerations, regulatory compliance, and data quality. Onix is an ML development company that helps businesses tailor machine learning technologies to their unique needs. We're here to help—just let us know about your product idea.
FAQ
What are the key benefits of integrating ML technology into businesses?
ML integration offers improved decision-making, enhanced customer experiences, predictive maintenance, optimized supply chain management, targeted marketing campaigns, and competitive advantage through data-driven insights.
What are the main challenges businesses may face when applying ML?
Businesses may encounter challenges such as high initial investment costs, data privacy and security concerns, biased outcomes, interpretability issues, and the need for specialized skills and expertise.
How have market leaders utilized ML successfully in their businesses?
Market leaders like Amazon, Google, and Netflix have employed ML to deliver personalized recommendations, language translation, image recognition, dynamic pricing, autonomous driving, and healthcare diagnostics, resulting in increased revenue and customer satisfaction.
Is ML integration a one-size-fits-all solution for businesses?
ML integration requires careful analysis and consideration of the business's needs and challenges. While it can provide substantial benefits, companies must evaluate whether the advantages outweigh the potential drawbacks and tailor ML implementation to suit their unique requirements.
What Role Does Machine Learning Play in Automating Business Tasks?
Machine learning (ML) automates repetitive, time-consuming tasks by analyzing data patterns and making real-time decisions.
This includes automating customer service with chatbots, streamlining data entry, and optimizing inventory management. ML can learn and improve over time, making processes more efficient and freeing up human resources for higher-value tasks.
How Can Small Businesses Benefit from Machine Learning Solutions?
Small businesses can leverage ML for affordable solutions that drive growth. For instance, ML-powered tools can help them personalize customer interactions, predict demand to manage inventory, and target marketing efforts more precisely.
This allows small businesses to improve customer experience, reduce costs, and make data-driven decisions, helping them compete more effectively with larger companies.
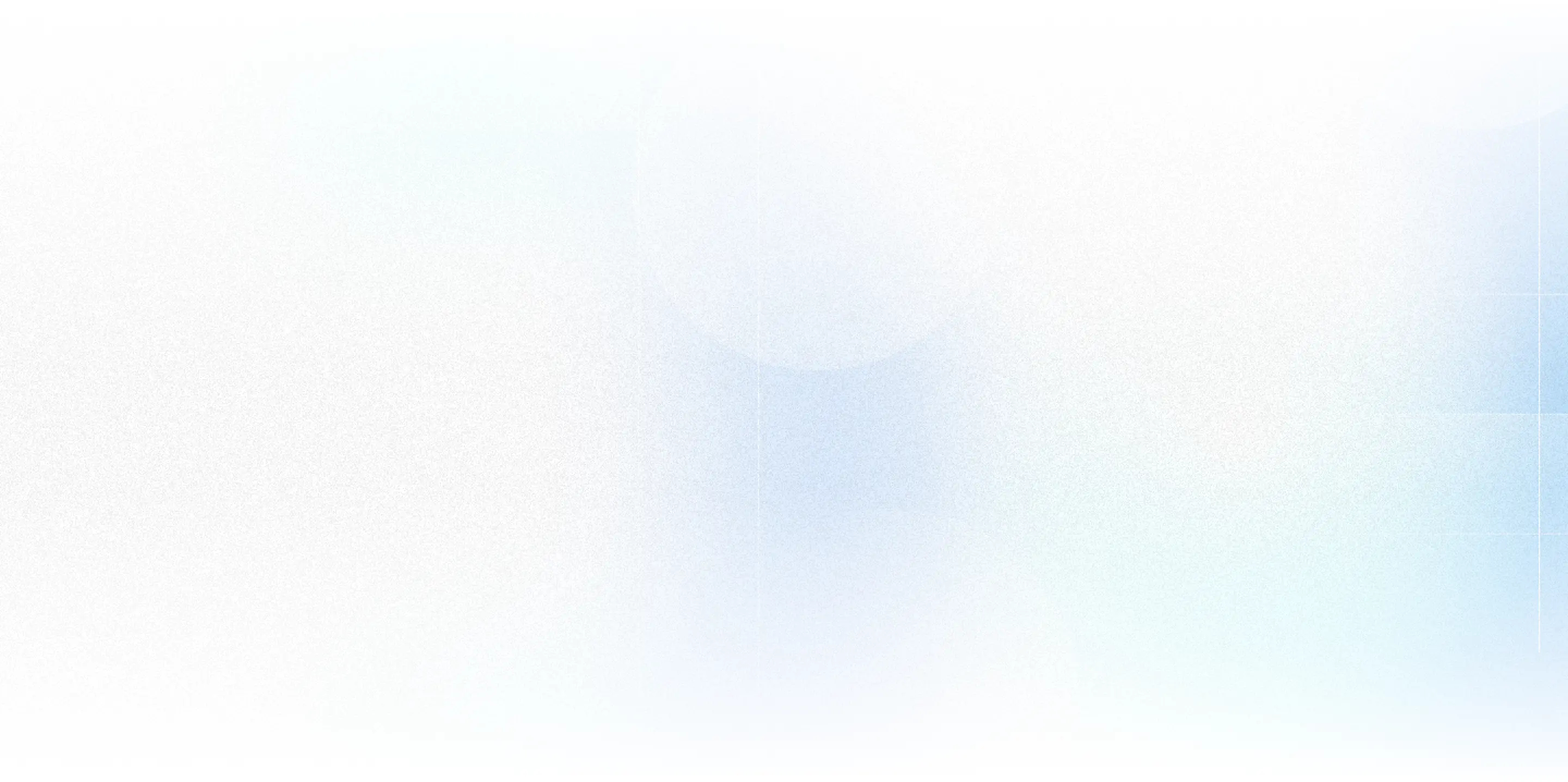
Never miss a new blog post from us!
Join us now and get your FREE copy of "Software Development Cost Estimation"!
This pricing guide is created to enhance transparency, empower you to make well-informed decisions, and alleviate any confusion associated with pricing. In this guide, you'll find:
Factors influencing pricing
Pricing by product
Pricing by engagement type
Price list for standard engagements
Customization options and pricing
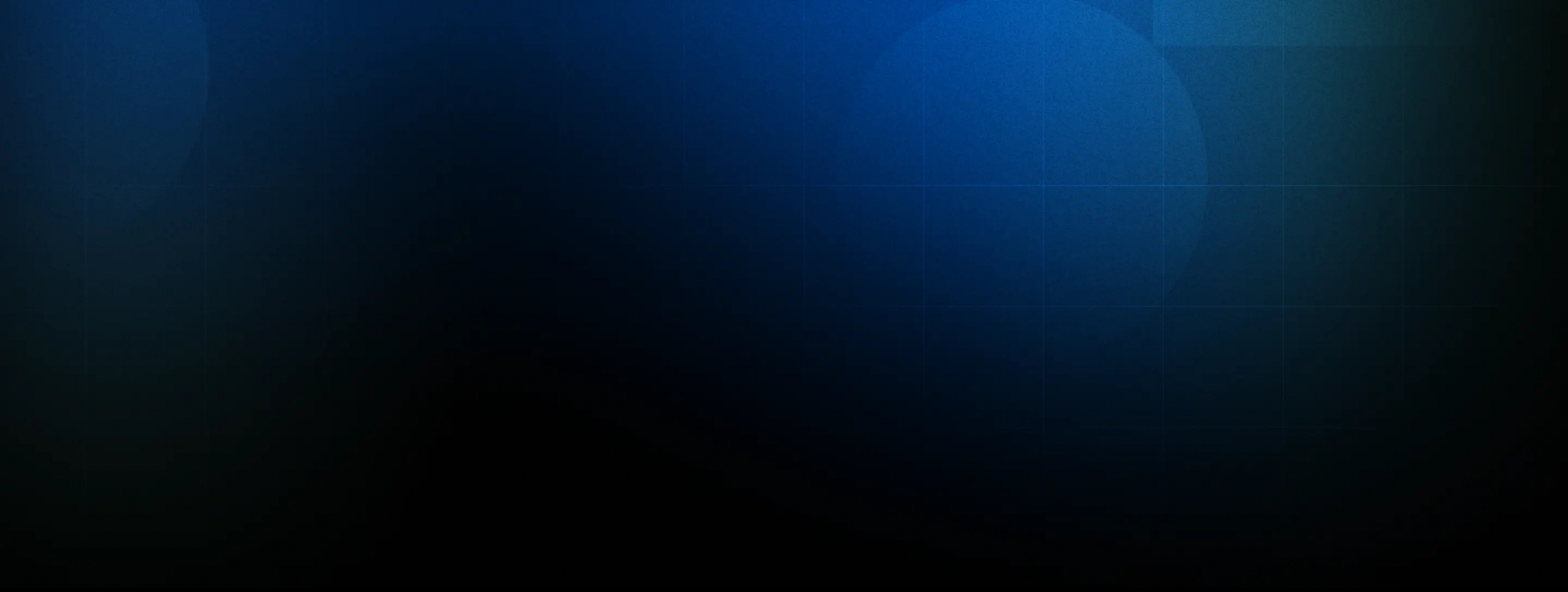